Drivers Of Land Use Change Map
BackgroundLand use/cover (LULC) change is a dynamic and complex process that can be caused by many interacting processes ranging from various natural factors to socioeconomic dynamics. It exerts a strong influence on the structure, functions and dynamics of most landscapes.
Monitoring and mapping of LULC dynamics are crucial as changes observed reflect the status of the environment and provide input parameters for optimum natural resources management and utilization. The objective of this study was to quantify the spatio-temporal LULC dynamics using satellite image coupled with local perceptions in the Gedalas watershed of the Blue Nile Basin, North Eastern Ethiopia. Maximum likelihood supervised image classification technique were employed to classify LULC categories. After ensuring acceptable accuracy value for each classified image, image differencing approach was used to detect and quantify LULC transitions of the area. Classification results were validated with the aid of field work, topographic maps, and high resolution Google earth images supplemented with other available thematic data sets. The resultsThe result demonstrated seven major LULC classes and the overall scenario presented by the study reveals that the watershed has experienced quite visible LULC transitions that seem to be continued in the future due to eternal anthropogenic activities and natural factors.
The study ascertain that though there was change in all land use types, the major change detected was a consistent expansion of farmland/settlements area mainly at the expense of Afro/sub Afro alpine vegetation areas. On the contrary, Afro/sub Afro alpine vegetation showed a consistent net loss of over the study of periods.
The findings also highlighted that transitions were ultimately driven by the interplay of biophysical, socioeconomic and institutional factors. Perceptions of the local communities on the LULC change substantially agree with data from satellite images. This implies that the ongoing rural land administration and natural resource conservation and management strategies could not effectively address the expansion of agricultural land towards fragile and marginal lands in the study area. Land resources, which are an integral component of the watershed ecosystem, are essential natural assets which provide social, economic and ecological functions to sustain livelihood of the inhabitants (Nunes and Auge ).
It is the platform on which human activities take place and also the source of materials needed for these activities (Briassoulis ). However, land is becoming a limited resource subject to competing demands and its various functions and services are seriously compromised by the problem of human induced land degradation (Gessesse et al. ).One of the prime prerequisites for better use and management of land resources is information on existing land use/cover patterns and changes in land use/cover through time (Anderson ). Spatial and temporal status of the land use/cover of a given area is an important parameter in understanding the interactions of the human activities with the environment (Anil et al.; Etefa et al. Land use and land cover patterns change in keeping with demands for natural resources (Anderson ). Studies have shown that although the evidences of land use/cover changes dates back many 1000 years, the recent rates, extents and intensities of human pressure on land and its scarce resources is more rapid and extensive than in any comparable period of time (Petit and Lambin; MEA; Ellis and Pontius ). This unprecedented human and environment interactions have been verified by LULC changes.Despite LULC change have social and economic benefits; this dynamic and complex process usually has an unintentional interlocked multidimensional consequence upon essential Earth’s ecosystem functions and services at both the small and large scales (Lambin et al.; Turner et al.; Lambin and Meyfroidt ).
For instance, changes in LULC has been shown to have negative impacts on biodiversity (Klenner et al. ), biogeochemical cycling and environmental degradation mainly due to exposure of soil to erosion forces (MEA; Meshesha et al.; Mwehia; Sewnet ), stream water quality (Uriarte et al.
); contribution to local and global climate change (Bringezu et al. ) and forest fragmentations (Ligdi et al.; Rands et al. ) which all have implications on the provisioning capacities of the watersheds. Although LULC change is global phenomenon, its nature and magnitude vary from area to areas. Its consequence is, however, strong particularly in fragile ecosystem and mountainous areas.Periodic LULC change monitoring is, therefore, an essential requirement for the assessment of ecosystem health and investigate factors responsible for triggering the dynamic processes and assess the environmental consequences of such changes (Lambin and Geist; Fichera et al. Moreover, information on LULC dynamics assists in monitoring environmental changes and developing effective land management and planning strategies at both national and local levels (Ellis and Pontius; Etefa et al.
).LULC change has become the focus of geographical research and discourse in Ethiopia for the last few decades (see Zeleke and Hurni; Garedew et al.; Kindu et al.; Temesgen et al.; Meshesha et al.; Desalegn et al.; Ariti et al.; Yesuf et al.; Demissie et al.; Etefa et al. ), but all of these studies were undertaken far from the relatively little known, sensitive and fragile areas of Beshillo catchments.

Hence, accurate and up-to-date spatio-temporal information on LULC dynamics, the driving forces and implications of these changes are urgently needed as an input parameter for planning site-specific sustainable land use and resource management practices in such overlooked place. The objective of this research is, therefore, to detect, quantify and map the LULC dynamics and trends as well as drivers and overall socioeconomic and environmental implications associated with these changes by integrating remote sensing information and the insights of the local communities.
Methods and materials. The study area falls into three agro-climatic zones: Temperate (Woina Dega), Cool (Dega) and Wurch (Afro alpine) zone (MOA ) (Fig.
The watershed is characterized by a bimodal pattern of rainfall with a 30 years mean annual rainfall of 930 mm. The highest average monthly rainfall (303 mm) was recorded in July; the lowest (10 mm) in December. The rainfall is variable from year to year, both in terms of intensity and distribution. The coefficient of variation of rainfall was calculated at 14.62% and standardize Rainfall Anomaly index also show variation over the period of observation which implying that there is inter annual rainfall variability in the area.
The monthly average minimum temperatures range between 6.4 and 8.6 °C while the maximum stretch between 17.9 and 21 °C. The mean annual temperature of the area is 13.6 °C (Fig.
Soil units found in the area are predominantly volcanic in origin. The major soil types of the watershed area are Leptosols, Cambisols, Regosols and Vertisols (Fig. Though the overall vegetation cover of the watershed are very poor, still there are various types of vegetation which range from scattered woodlots to Afro alpine types. Aside from the patches of eucalyptus trees planted by farmers and government initiated programs; there are a few dispersed indigenous tree species, shrubs and afro alpine species unique to extreme highland parts of the watershed. Fig. 4Dominant soils types of the watershedAt the time of this study, the watershed was occupied by a total population of approximately above thirty-five thousands (Estimation from TWARDO ), which makes a rural crude population density of close to 147 persons per square kilometer; considerably higher than the regional average of 111 persons/km 2 (CSA ). Agriculture is the main economic activity and source of livelihood.
The farming system is almost entirely rain-fed and involves the integration of crop cultivation and livestock rearing that is carried on at subsistence level. Soil tillage is carried out with traditional ard plough (locally called Maresha) pulled by a pair of oxen. The frequency of tillage ranges from 1 to 3 per cropping season depending on crop types.
The major crops of the area are cereal crops, including sorghum ( Sorghum bicolor), Oats ( Avena Sativa), teff ( Eragrostis tef), maize ( Zea mays), barley ( Hordeum vulgare), senar/engedo ( Avena sativa) and wheat ( Triticum vulgare). Barley and senar/engedo are the dominant crops cultivated in the Wurch area of the watershed and sometimes is rotated with wheat.Other major crops are pulses such as Chickpea ( Cicer arietinum), vetch ( Vicia sativa), Field Pea ( Pisum sativum), and Faba Bean ( Vicia faba).
Programma Per Scaricare Giochi Ps2 Iso Emulator Download Giochi. Roms Isos PSX, PS1, PS2, PSP, Arcade, NDS, 3DS, Wii, Gamecube,. Programma Per Scaricare Giochi Ps2 Iso Download. The compatibility rates for the emulator boast over 80% of all PS2 games as playable. DirectX compatible Sound Card. CD-ROM or DVD-ROM Drive. DirectX 7.0. I Giochi disponibili sono per PC, Xbox 360, PS2, PS3, PSP, NDS e WII. Play Console Classics on your Computer - Wii ISOs, 3DS ROMs and More! Scarica giochi e programmi gratis. Siti torrent internazionali per scaricare i giochi: 1. Download giochi wii iso ita gratis These flash cards enable you to play homebrew. Installation Steps. Insert the CentOS 7 CD-ROM in the CD-ROM drive. Power on the. Per Programma Per Scaricare Giochi Ps2 Iso Roms. Programma Per Scaricare Giochi Ps2 Iso Roms. July 1, 2017. Share on Facebook. Share on Twitter. Please reload. Black Facebook Icon Black Twitter Icon.
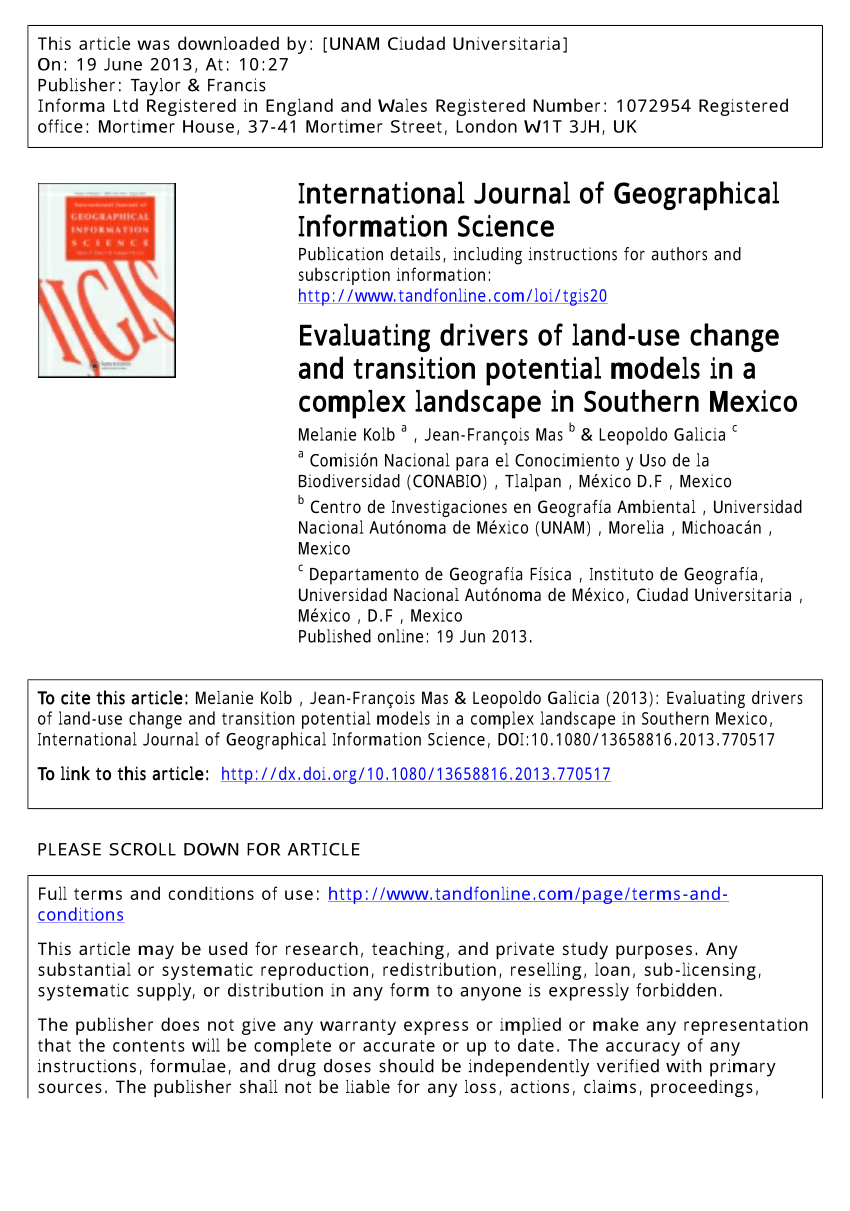
Sorghum ( Hordeum vulgare) and Maiz ( Zea mays) are also produced on the lower reach of the watershed. Four sets of digital satellite imageries such as Landsat MSS, TM, ETM+, and Landsat 8 of the year 1973, 1986, 2001 and 2017, respectively were used to examine spatio-temporal LULC dynamics of the watershed (Fig.
The selection of these satellite images was entirely based on the quality (e.g. Cloud free) and long time series availability, correspondence with years of major regime/policy changes and/or events in the country, purpose of the question to be answered, and suitability of the seasons for collecting socioeconomic field data. The Landsat MSS 1973 image had a resolution of 79 m; while the Landsat TM 1986, Landsat ETM+ 2001 and landsat 8 images have a resolution of 30 m (Table ).
Land Use Map Indiana
All these terrain-corrected and radiometrically calibrated Landsat images were freely accessed and downloaded from the online archive of the United State Geological Survey (USGS) Centre for Earth Resources Observation Systems (EROS) available at ( ). It would have been better to start with medium resolution images such as Landsat TM images for the study but since there were no such images in the 1970s; a lower resolution Landsat MSS 1973 image was taken as reference year for this study. During the acquisition of satellite image and associated metadata, information pertaining to platform, sensor calibration, projection, coverage, resolution, cloud covers and other relevant information were taken into account. The effects of solar illumination angle induced by the rugged terrain nature of watershed which may cause different reflectance for the same cover type were considered.
TM thematic mapper, ETM + enhanced thematic mapper plus, OLI operational land imager, TIRS thermal infrared sensorThe acquisition dates of images were made as close each other as possible to minimize troubles related to seasonal variations. Moreover, dry and clear sky months (December to January) were preferred to mitigate the effects of atmospheric conditions that blight the quality of optical remote sensing imagery. Since these months are seasons of harvesting, they are relatively ideal to easily identify each land use/cover types.All imageries were projected to Universal Transverse Mercator (UTM) zone 37 N using World Geodetic System (WGS) datum (WGS1984UTMZone37N) to ensure consistency between datasets during analysis. The are displayed in the table below. Supplementary dataTo validate classified images accuracy and further investigate driving forces and associated impacts of LULC changes, supplementary data, such as DEM, topographical maps and socio-economic data were collected from various sources. The Shuttle Radar Topography Mission (SRTM) DEM (30 m spatial resolution) was obtained from the United State Geological Survey (USGS) ( ). A digital elevation model was used for extracting slope properties, prepare topographic map, delineate and identify sub watershed areas, among others.
Different Land Use Categories
The high-spatial resolution Google Earth was employed to collect the training and testing samples points for land use/cover classification and validation. The 1993 edition three sheets of (1039 A1, 1139 C3 and 1139C4) 1:50,000 scale and 40 m contour interval digital Topographic map were purchased from Ethiopian mapping agency (EMA) and used for geo-referencing of satellite images, preparation of the base maps and for LULC class verification and interpretation.Socio-economic data were collected from the community through a series of questionnaire, key informant interview, focus group discussions which was facilitated by local development agents with the presence of the author and complemented by onsite field observations. The data type captured from local knowledge/experience were the nature of LULC change over time, perceived drivers of such changes and site-specific management efforts. Additional information supporting the interpretations, including policy documents, proclamations, implementation guidelines and statistical reports were obtained from national and regional government’s offices and official websites. Data analysis was done using SPSS v 22 and MS Excel 2010 Packages while the Satellite image analysis was performed with the help of ArcGIS 10.3 and ERDAS IMAGINE 2014 Softwares. Data verification and error controlData verification and error control have been given special attention in this study. To identify and interpret the main LULC types, field visit and observations were carried out at different times in the watershed.
During the field survey, data on the historical and present LULC types were collected and localized by using GPS (GARMIN-60). Moreover, these reference areas were documented by photographic images (using digital camera) to strengthen the reliability of the data gathered. The GCPs information for the LULC types of the past were identified from elderly people, author’s prior knowledge and knowledgeable assistants from related government departments, such as agriculture and Natural resource offices of Tenta Woreda. Digital image processing and land use/cover classificationsAfter selection and acquisition of appropriate satellite imagery in terms of coverage, resolution and accompanying metadata from the sources, an intensive preprocessing, such as re-sampling and layer stacking of the required bands were performed.
To ensure image pixels uniformity, Landsat MSS 1973 image was re-sampled to 30 m pixels using the nearest neighborhood method (Johnson ). Then, the study area was extracted from the images using Subset tools in Erdas Imagine 2014. After creating subset images covering the watershed and checking the quality of the image, a classification scheme was developed to derive various LULC classes of the watershed.To classify LULC categories, more than three hundred randomly distributed training sets of data, varying in size from 20 to 60 based on area coverage, were employed to locate training pixels for each land use/cover category and study periods. The training sites for recent images were generated by GPS reading supported by high resolution Google earth images while the training sites for older historical images were assigned with the aid of raw images visual interpretation, topographic maps, old black and white ground photographs of some sites within the study area, supplemented by local elder’s information and author’s prior knowledge. This approach was used by other researchers in their study of LULC change in Ethiopian highlands (Kindu et al., Demissie et al. The training signatures were then evaluated for class separability between LULC classes.
In the evaluation stage, signature editions were done by deleting, merging or renaming until the most satisfactory result achieved.Finally, supervised classification and visual interpretation technique were applied for all the images, following Maximum Likelihood classification algorithm and change detection comparison strategies. Authors prefer to use the Maximum Likelihood algorithm with the assumption that it minimises classification error for classes because this approach incorporates both class covariance matrices and mean vectors as signatures, and merging of constituent spectral class signatures to obtain those for rationalized classes (Jensen and Lulla; Foody; Otukei and Blaschke; Kantakumar and Neelamsetti ).In the process of classifications, some LULC units were misclassified to other classes. For instance, bare lands were misclassified to the farmland/settlements class.
This happens due to the fact that some bare land’s spectral properties appeared similar to that of harvested croplands which bring difficulties in distinguishing them during image acquisition. To improve classification accuracy and reduce misclassifications, recoding techniques were applied to clean misclassified ones using ERDAS software through visual inspections. To simplify and reduce the number of LULC classes, some related LULC classes were merged together into one class. For instance, Croplands and settlements were aggregated as farmlands/Settlements as it was difficult to identify the dispersed rural settlements, where in many cases hamlets are surrounded by farmlands, into separate land use/cover types. Similarly, due to the intermingled nature of shrub and bush lands, it was difficult to distinguish these two categories and hence was labeled as shrub/bush lands to report the LULC study results (Table ). Nonetheless, the authors realized that combining the different LULC classes could hidden differences among them and may exert its impact on the quality of the outputs (Misana et al. For nomenclature purpose, LULC classification types commonly used for Ethiopia were referred and adopted for ease comparison of results.
S/NLand use/cover classes aDescription1Woodlands/plantationsAreas covered with intricate mixture of small trees and bushes. These categories also incorporate Eucalyptus woodlots and/or other remnant woody plantations found mainly in the form of farm boundary, in and around scared sites2Shrub/bush landsAreas covered with woody shrubs, thorny bushes and scattered or patches of various species of small trees, usually found along banks of streams, rugged landscapes and escarpments.

Some of these land cover is used for communal grazing and browsing purpose. Then, the overall accuracy, Kappa coefficient, producer’s accuracy and user’s accuracy were calculated from the error matrix (Foody; Fan et al.; Congalton and Green ). The overall classification accuracy was computed by dividing the number of correct values in the diagonals of the matrix to total number of values taken as a reference point; producer’s accuracy was derived by dividing the number of correct pixels in one class divided by the total number of pixels as derived from reference data; user’s accuracy was calculated by dividing correct classified pixels by the total number of pixels and Kappa coefficient, which measures the agreement between the classification map and the reference data, were calculated as per Bishop and Fienberg ( ) (Eq. (1)where K is Kappa coefficient, r is the number of rows in the matrix, x ii is the number of observations in row i and column i (the diagonal elements), x i+ are the marginal totals of row i, x + i are the marginal totals column i, and N is the total number of observations.
Change detection and analysisThe changes in LULC that had occurred in the watershed over the period of study were detected through post-classification comparison approach (Singh; Fan et al.; Chen et al. Post classification comparison (map-to-map comparison) methods were preferred in this study for the number of reasons. Firstly, using this method minimizes the problems associated with multi-temporal images recorded under different atmospheric and environmental conditions and by different sensors, (Singh; Yuan et al. Secondly, this methods provide the extent and nature of ‘from-to’ change information (Jensen ).
Based on this ground, four LULC maps were compiled to display the type of LULC classes and compare between the classified images. Then the whole study period (1973–2017) was classified into four sub-periods (1973 to 1986; 1986 to 2001; 2001 to 2017; and 1973–2017 which includes the entire 43 years of study periods). Then, paired overlay was performed through spatial analysis in GIS in order to detect, compare, and analyze patterns and directions of changes and to quantify persistence, gains, losses, total change, net change, and swapping of LULC occurred during the time period considered in the watershed (Pontius et al. Conversion matrix was used to distinguish the changes of each category at the expense of others and its general structure follows the format displayed on Table.
The rows display the categories of time 1 (initial Time), and the columns display the categories of time 2 (subsequent time). Entries on the diagonal (that is, P jj) indicate the amount of land use/cover category which remained persistence of class j between the time period and used to calculate the gains and the losses of land use/cover classes while the off-diagonal entries show the size of the area that transitioned from category “ i” to a different category “ j” during the time interval (Aldwaik and Pontius ). For ease of reference, the equations and notation used to compute various components are presented as follows. Time 1Time 2Total time 1LossLULC 1LULC 2LULC 3LULC 4LULC 5LULC 6LULC 7LULC 1P11P12P13P14P15P16P17P1+P1+ − P11LULC 2P21P22P23P24P25P26P27P2+P2+ − P22LULC 3P31P32P33P34P35P36P37P3+P3+ − P33LULC 4P41P42P43P44P45P46P47P4+P4+ − P44LULC 5P51P52P53P54P55P56P57P5+P5+ − P55LULC6P61P62P63P64P65P66P67P6+P6+ − P66LULC 7P71P72P73P74P75P76P77P7+P7+ − P77Total time 2P+ 1P+ 2P+ 3P+ 4P+ 5P+ 6P+ 71GainP+ 1 − P11P+ 2 − P22P+ 3 − P33P+ 4 − P44P+ 5 − P55P+ 6 − P66P+ 7 − p77. (10)If the net change is zero (implying gain is equal to loss), then the swap is twice the loss or gain.The exposure of each land use/cover classes for a change were assessed using the loss to persistence ratio (Lp = loss/persistence) which assesses the vulnerability of a land use/cover classes for a change; gain to persistence ratio (Gp = gain/persistence) which evaluates the gain of a land use/cover in comparison to its time 1 size, net change to persistence ratio (Np = net change/persistence) (Braimoh; Ouedraogo et al. ).Values of Gp and Lp greater than one imply that a given land use/cover class has a higher probability to change to other land use/cover class than to persist in its current condition (Braimoh ).
If the value of Np was negative, the land use/cover class would have a higher probability to lose area to other land use/cover classes than to gain from them. Finally, two sorts of data were generated; namely, four land use/cover maps, which illustrates the changes in a spatial context and various tables which exhibit the amount of areas for each LULC categories and a cross-tabulation matrix which demonstrate LULC transition from category to category at different study periods. Moreover, bar graphs and tables were used to display quantitative land use/cover class as a function of topography (i.e. Altitude, and slope categories). The overall methodological flow chart showing the sequence of data acquisition, image classification and analysis is depicted in Fig. The spatio-temporal quantity of LULC types of each category was analyzed in terms of total area and percentage for each study periods (Table, Fig. The result indicated proportion of each LULC classes varied considerably on different dates considered.
In 1973, the dominant LULC types in the watershed were farmlands/settlements accounting for more than 50% of the total area followed by Afro/Sub afro alpine vegetation (19.8%), grass lands (16.3%), and shrub/bush lands (10.8%). Bare lands, Woodlands/plantations, and Water courses/beds accounted the smallest proportion of the watershed. Though the extent varied among land use classes, the order of proportion occupied by the LULC types in the study area remained the same in 1986. In 2001, farmlands and settlements were still the dominant category (48.4%), followed by Afro/Sub afro alpine vegetation (19%) but the ranks of shrub/bush lands (14.8%), and grass lands (12.8%) were reversed. These orders continued for 2017 LULC distributions.
The results showed that farmlands/settlements were/are remained by far the dominant LULC of the watershed for the last 43 years in the watershed. Land use/cover type012017Area (ha)% of totalArea (ha)% of totalArea (ha)% of totalArea (ha)% of totalAfro/sub afro alpine vegetation4599.04351314.66Bare lands3971.602.176132.56Farmlands/settlements12,81553.46Grasslands3702.89304012.68Shrub/bush lands2244.80301012.56Water courses/beds1230.10.42910.38Woodlands/plantations17.883.70Total23,9,9,9,970100.00. The overall classification accuracy report of 97, 89 and 96% were attained for the 1986, 2001 and 2017 classified images, respectively. Since the values falls above the cut point of the standard overall classification accuracy level of 85% (Anderson; Congalton and Green ) with no class less than 70% (Thomlinson et al. ), we can conclude that there is an acceptable agreement between the classified image and the ground reality it represents.
A kappa coefficient result was found to be 0.96, 0.78, and 0.94, for years 1986, 2001 and 2017 respectively (Table ). The results showed a strong agreement for each of the three classified images (Lea and Curtis ). UA user’s accuracies, PA producer’s accuracies Land use/cover changes and its driving forces in Gedalas watershed (1973–2017)The LUC changes were categorized into four periods 1973–1986 (first period), 1986–2001 (second period), 2001–2017 (third period) and 1973–2017 (whole period). Change detection diagnostics showed that in the first study period (1973–1986), total area of farmlands/settlements decreased from 50.2 percent to 42.7 percent irrespective of gains made from other LULC types, but then it showed an overall increasing trend throughout the whole study period with the highest change rate being observed in the period from 2001 to 2017 (Table ). The reduction in the first study period was most likely due to abandonment of farmlands by farmers for reasons of massive rural out-migration and resettlement program of the government to mitigate severe land degradation and historic drought episodes of 1984/85 which had a devastating impact on human population and livestock resources of Wello (North Eastern Ethiopia) in general the study area in particular.
Such abandoned farmlands were presumably taken over by grass and regenerated into shrub/bush covers hence the observed increase in this two-land use/cover types in this period. For example, the famine of Wello caused by drought of 1984/85 is still remains as tragic memory to the world community.
The nexus of population growth and changing diets has increased the demands placed on agriculture to supply food for human consumption, animal feed and fuel. Rising incomes lead to dietary changes, from staple crops, towards commodities with greater land requirements, e.g. Meat and dairy products. Despite yield improvements partially offsetting increases in demand, agricultural land has still been expanding, causing potential harm to ecosystems, e.g.
Through deforestation. We use country-level panel data (1961–2011) to allocate the land areas used to produce food for human consumption, waste and biofuels, and to attribute the food production area changes to diet, population and yields drivers. The results show that the production of animal products dominates agricultural land use and land use change over the 50-year period, accounting for 65% of land use change. The rate of extensification of animal production was found to have reduced more recently, principally due to the smaller effect of population growth. The area used for bioenergy was shown to be relatively small, but formed a substantial contribution (36%) to net agricultural expansion in the most recent period.
Nevertheless, in comparison to dietary shifts in animal products, bioenergy accounted for less than a tenth of the increase in demand for agricultural land. Population expansion has been the largest driver for agricultural land use change, but dietary changes are a significant and growing driver. China was a notable exception, where dietary transitions dominate food consumption changes, due to rapidly rising incomes. This suggests that future dietary changes will become the principal driver for land use change, pointing to the potential need for demand-side measures to regulate agricultural expansion. Previous article in issue. Next article in issue.